Is AI the silver bullet that will solve all your problems? Right now, it seems unlikely! But Hive members are putting Artificial Intelligence to use right now, getting AI to supercharge productivity, cut timelines and tackle complex problems within their businesses. From those just getting started to retailers who are embedding AI into applications, find out how Hive members are using AI to reach their goals quicker and more efficiently
In a nutshell…
- It’s evolving teams. The advent of Copilot is steering structural shifts, necessitating a greater presence of Business Analysts within team ranks
- Use AI as a strategic assistant. AI is positioned to augment human endeavors, not replace them
- Take caution with LLMs. Large Language Models are akin to expansive rear-view mirrors—to be treated with caution
- Data’s paramount role. The efficacy of AI is intricately tied to the quality and source of data
- Human oversight is needed for brand protection. In instances where AI-generated content interfaces with customers, a human review is indispensable for safeguarding brand integrity
A closer look…
AI in action
AI is a dynamic feedback loop between humans and machines. The core message for Hive members? AI is not a force designed to replace humans but rather to assist and empower them. The cautionary tale of a recent chatbot error which was widely shared (and derided) on Twitter serves as a stark reminder of the imperative to keep a distance between experimental AI initiatives and the direct customer exposure to preserve brand equity.
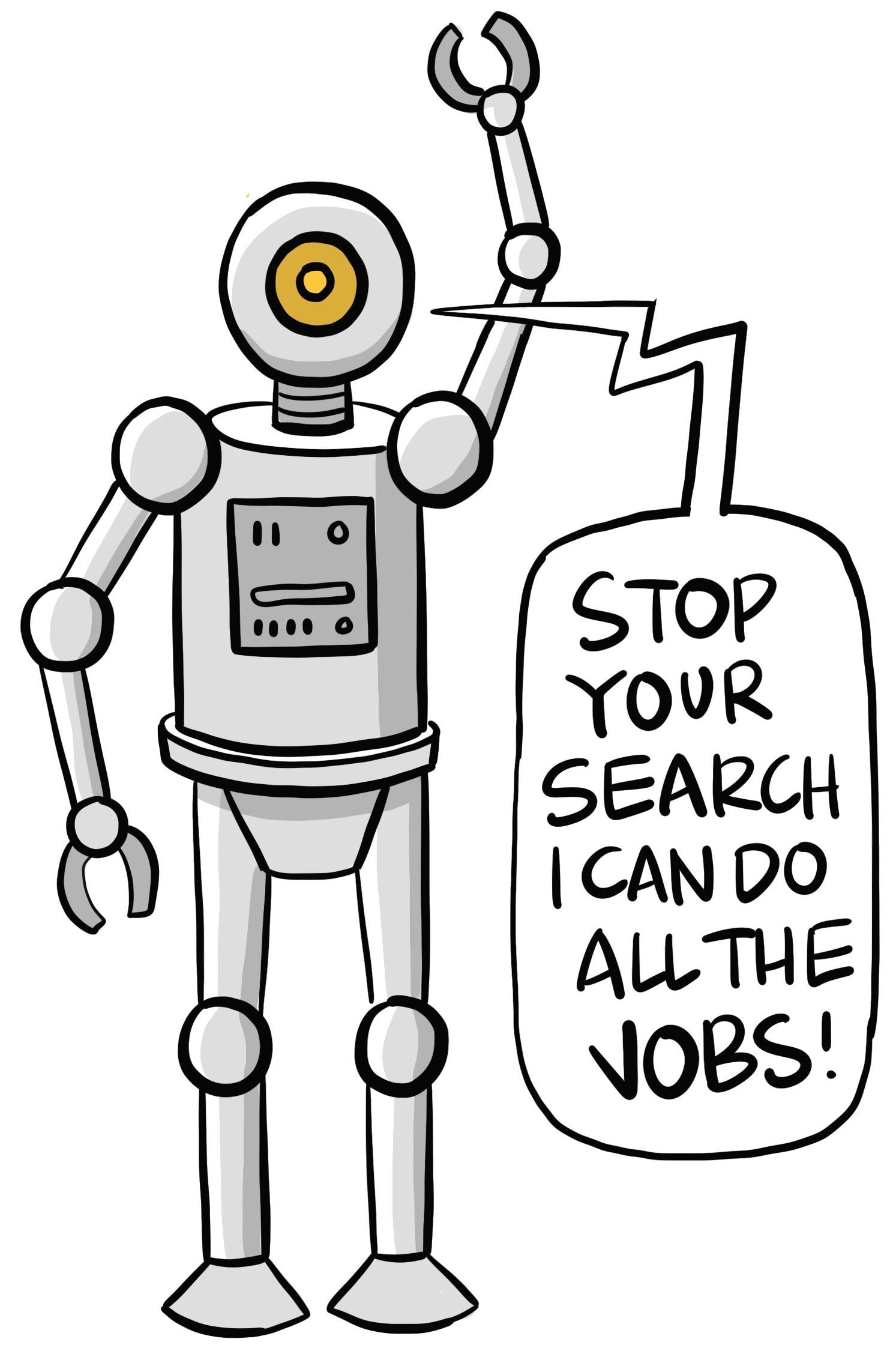
Who’s setting a good example? Octopus and leaders in the insurance sector demonstrate AI’s ability to navigate complex tasks, providing indispensable support to human agents rather than superseding them. When it comes to coding, some Hive members anticipate software engineers becoming ‘prompt engineers’ and AI boosting productivity by up to 500%, yet the need for the ‘human in the loop’ is still vital. The transformative journey extends into customer experience, where AI has been used across our membership to speed up processes and enhance transparency through linkage with internal data sources.
Still to do…
One critical factor is the need to address biases within AI algorithms. Ensuring alignment with different language patterns, particularly in regions like Asia, remains a challenge for AI. When it comes to deciding whether building or buying AI solutions is best, it’s clear that for Large Language Models (LLM), the bigger players are pulling away from smaller competitors at the moment and that tech giant firepower is getting results.
Forecasting improvements are still a persistent challenge, as LLMs provide information, yet the crux lies in human insights to make informed decisions. The main lesson here is that well-framed exam questions are pivotal in justifying continued investments in AI.